Benedict Evans: ‘AI will just be software...unless it kills us all’ /
Tech and media analyst Benedict Evans on the artificial intelligence ‘platform shift’ and why generative AI is giving people ‘infinite interns’.
James Swift
/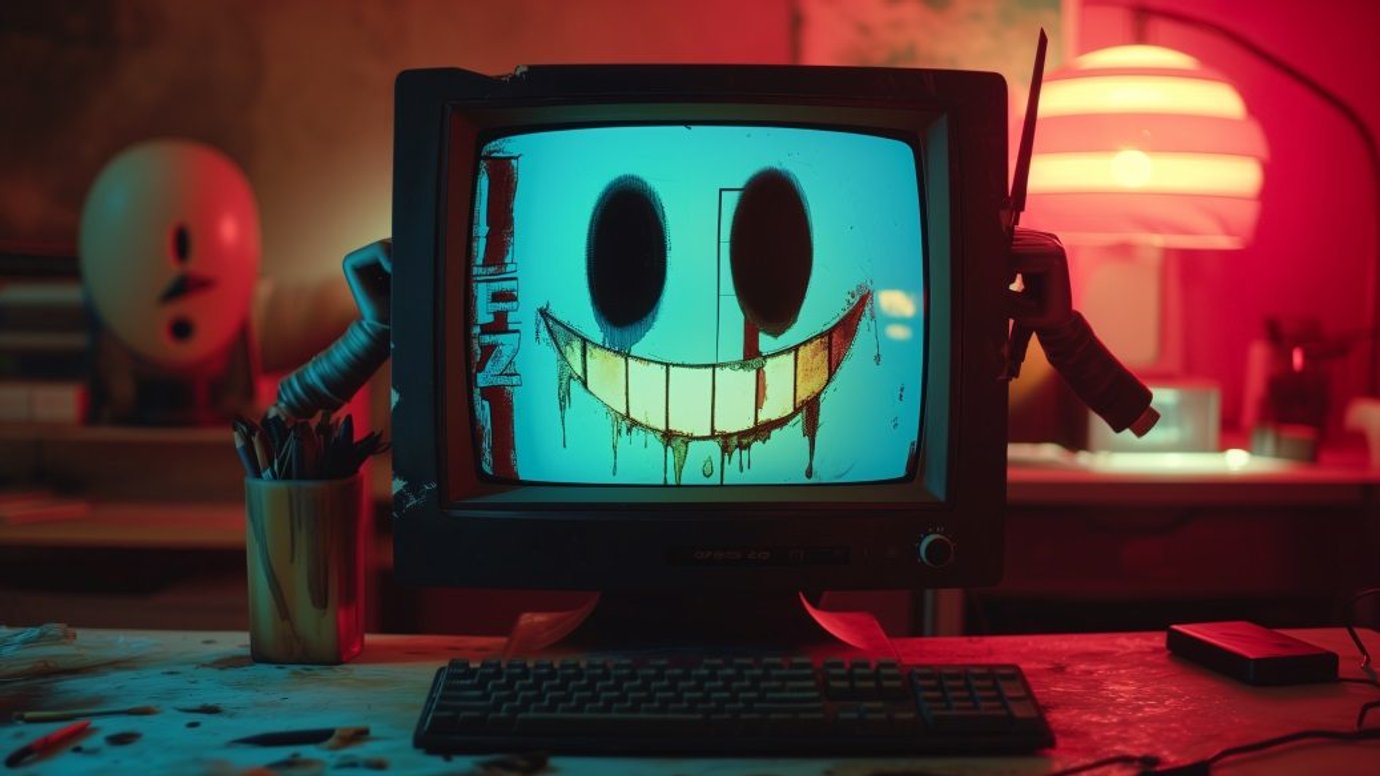
No one knows how artificial intelligence will ultimately change the way we work and live, and you should be suspicious of anyone who says they do. But some people — though probably far fewer than claim to — at least understand what the technology is capable of today. And an even smaller pool of people have decades of experience analysing the tech and media industries to draw on to put that understanding into perspective, and come up with some practical and interesting insights into AI.
Benedict Evans is one of those people. He is an independent analyst — and former partner at Silicon Valley venture capital firm Andreessen Horowitz — who has created a reputation for himself as one of the most knowledgeable and level-headed tech commentators around through his newsletter, which goes out to more than 175,000 subscribers.
Every year, Evans publishes a presentation that explains what’s happening in tech and what direction it is headed. His most recent presentation was called ‘AI, and everything else’. And just after the New Year, we got to talk to Evans over Zoom, to get the grown-up take on the latest tech innovation.
In terms of the pace of development and adoption, what previous technology does AI remind you of, and what does this tell you about its likely trajectory?
This looks like a platform shift. The tech industry has a platform shift sort of every 10 to 15 years. We went from the PC to the web, and then we went from the web to smartphones. And now we go to generative AI.
Benedict Evans, Analyst
Parallel [to that], we also had the adoption of open source 20 years ago and the adoption of machine learning 10 years ago. And there’s always two or three processes as these arrive. There’s a process where we try and work out what this is, what’s the right way to understand it, what’s it useful for, how does it get absorbed, how does it turn into something that’s always been there.
There’s also a process where the incumbents try and make the new thing a feature, and meanwhile, people create startups to unbundle aspects of the incumbents. And then people can work out things that wouldn’t have been possible with the old thing. If you think about mobile, you start out by taking your desktop website and kind of squashing it into an app. But then you also get things that you can only do once you have a camera and GPS. So, step one is Addison Lee having a cab booking website, step two is Uber.
What stage of that curve or process are we in at the moment?
It's happening much more quickly than previous shifts because for users, [generative AI] is just a website. You don’t need to wait for consumers to buy new devices, you don’t need to deploy huge amounts of new infrastructure because the data’s all in the cloud already. This is a new thing that’s been built on data infrastructure that was built in the last 10 years. So you have this sudden moment when OpenAI announced this thing [ChatGPT] and suddenly they’ve got 100 million users and something like a billion dollars of revenue. So you’ve got this great rushing noise as everyone goes to deploy it.
But you then have a moment where you think, ‘Okay, we’ve all tried this, but how do we understand what we do with it?’ Step one is everyone in an ad agency types some stuff into Midjourney and goes, ‘that’s fucking cool!’. And then you think, ‘Well, yeah, but we can’t just forward the client brief to Midjourney and then forward the Midjourney output to the printing company.’ A little bit more has to happen in the middle of that. It’s the same thing for a law firm or an engineering company. It takes time to work out how you turn this from an amazing demo into a product.
So that’s where we’re at now? We’re waiting for people to take this technology and create something with it?
I think so, yes. And there’s the stuff that works immediately. I’m sure you’ve had this experience of, ‘I’ve got to do a user survey by tomorrow morning, the associate hasn’t done it and it’s 11 o'clock at night. Fuck, I’ve got to write 20 questions.’ And you go to ChatGPT and it gives you 50, and you pick 10 that are good and then you think of another 10.
So, on the one side, you’ve got these code-writing assistants where the mistakes are obvious and easy, and it saves a lot of time. And the other side is brainstorming, where I want 10 sketches of this or 50 ideas for a headline, and then you pick the ones that work. That’s the obvious, early thing, but it’s a bit like printing out your emails — you take the new tool and you force it to fit existing tasks. Then, over time, you work out how we actually change the way we work in order to reflect that this thing exists.
I wasn’t even going to ask you what you thought those later uses might be because I take it no one knows yet?
There are conceptual ways that you can build towards this. I always remember when I was a mobile analyst in the mid 2000s. Every conference you went to, somebody would say that in the future, you’ll be walking past Starbucks and your phone will know where you are, and you’ll get a coupon by SMS for a free coffee. It was kind of a joke — people would say it, just to repeat the joke. But I don’t think that happens even now. And, of course, [if it did], it wouldn’t be with SMS. Meanwhile, people were making models predicting that telcos would be making money from it. They would charge you for every location lookup because phones didn’t have GPS then, so you were doing it with a cellular triangulation off the cellular towers. Meanwhile, nobody looked at taxis and said, ‘Hey, what if you put GPS in the taxis? And what if you had an app that had GPS, then you could completely change how it actually works?’ And you can kind of run this model over and over and over again.
When you talk about AI as a platform shift, is that the bear case for the technology or the bull case? Or is it somewhere in between?
I think the base case is that you can have this very schematic model that says there are PCs and the web, and then there’s smartphones, but the reality is that there’s a bunch of different things going on at any given time. So there are PCs, but there’s also the shift from mainframes to client servers. And a shift from IBM to Oracle. And then there’s the adoption of open source, the deployment of the cloud and machine learning, and these all overlap and play off each other. So it’s not a binary thing. That’s what I’m getting at. Is this [AI] what every new software company will get built around in the next couple of years? Yes. On the other hand, guess what? Microsoft is still around. IBM is still around. Google sort of turned into Microsoft, and Microsoft sort of turned into IBM. Mobile happened, and that turned out to be great for Facebook. So these things are never neat and tidy.
You’ve previously said there are no or few answers about AI at the moment. What then are the most pertinent questions?
I think that there are hierarchies of questions. So from a science and engineering perspective, how much better do the models get? Are we on an S-curve? Will it flatten out at a given level or will it accelerate upwards? How general purpose are they? Obviously the new thing is multimodal models that can do images and text and video and audio and generate music, and so on. Does this evolve to look something like the last wave of machine learning? Or indeed, like databases? If you were to ask today, ‘how many databases are there?’ That would be a ridiculous question. It's like saying how many spreadsheets are there? You don’t even know how many databases are inside your company — and no one cares.
It’s not clear that [AI] will look quite like that because the whole point is massive scale. But there’s one extreme in which there are a handful of giant, capable, capital intensive, expensive and very general models [...] and everything else plugs into that. And then there’s another extreme, which says it looks like spreadsheets or databases.
It’s not clear that it will look quite like spreadsheets or databases, but you’re already seeing this sort of proliferation of models. And a huge amount of the science is into, ‘Well, we can’t add two more orders of magnitude more data to this stuff, and meanwhile, it’s fucking expensive. So how do we make them smaller, more efficient, more specialised, more fragmented? Can you put models onto mobile devices?’ What’s the next step after the one giant model?
I think those are the basic science questions. Obviously, one corner of that is the AGI [artificial general intelligence] conversation, which reminds me a little bit of the crypto conversation — a lot of very cultish people talking to each other and talking as though this is all obvious and self evident, and everybody else is an idiot, and the rest of us looking at them and going, ‘Mmmmm, I don’t think so’.
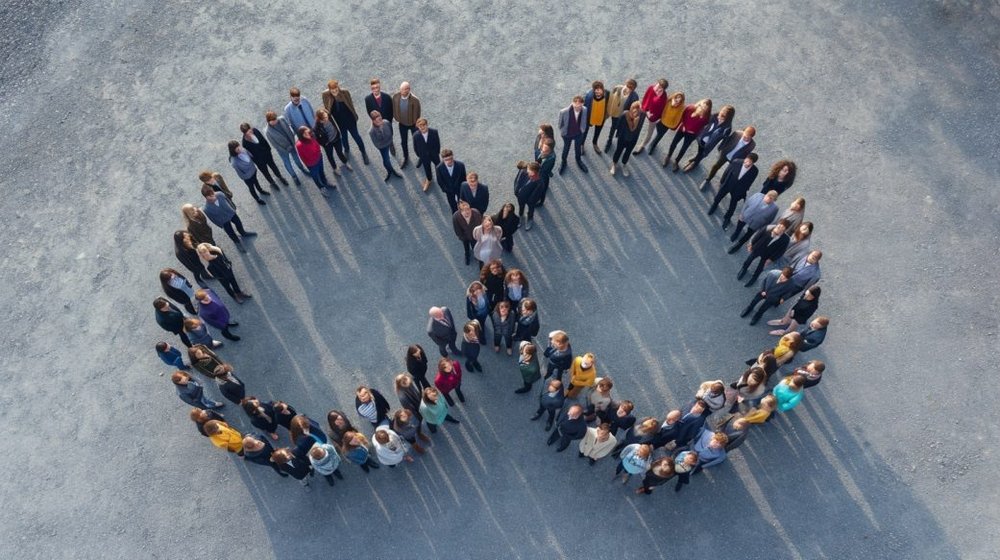
I think you’ve previously said that generative AI is good at things that computers are bad at, but bad at things computers are good at. What does that mean?
I saw someone say that online, so it’s not my quote. But yeah, the analogy I was using for the last wave of machine learning was to think of this as giving you infinite interns. Say you would like to listen to every call coming into the call centre and tell me if the customer’s angry. An intern could do that. A dog could do that. You could train a dog to do that, almost literally [...] The caveat is that you have to check it because there’s an error rate.
This is kind of the same thing now [with machine learning]. Imagine you had a million interns, what are you going to do? Get them to give you a thousand ideas for slogans in 10 minutes. You’ll have to check them, but it’s still fantastically useful to have a bunch of interns that can give you a thousand ideas in 10 minutes. You can’t just give that to the client, but it’s still fantastically useful to have it.
I might be paraphrasing some of your earlier work again here, but if generative AI is best used for tasks where either the correct answer doesn’t exist or doesn’t matter, does that mean that its uses are much more suited to tasks around imagination and creativity?
I don’t think so, no. Maybe something I should have drawn out explicitly earlier [is that] because these are probabilistic systems, they’re not databases and they’re not consistent. They will not give you exactly the same answer every time. And they’re not information retrieval systems, which is what we’ve all suddenly discovered. When you ask it about something you don’t know anything about, it looks completely convincing. Ask it something you know a lot about, then you will realise all of this looks like the right answer, but it’s not the right answer.
So the question is, where is that a meaningful statement? If I say, ‘give me 500 ideas for a slogan for a new brand doing X’, there’s better and worse answers to that, but there isn’t a wrong answer.
There are some questions where you want a deterministic answer, and you see where it matches; like with code, you can see when that’s wrong. There’s other kinds of questions where a probabilistic answer is what you want. What kinds of slogans would people probably give for a new brand in X field? That doesn’t have a wrong answer. I think where we progress is, how close can you get those probabilistic answers to deterministic answers? And how can you manage that? Can you give sources, can you indicate certainty, can you say this is absolutely correct? Does the model get good enough that it doesn’t matter?
There is this whole sort of drunken philosophy, graduate student conversation of how maybe people don’t have general intelligence, either. Maybe we’re just giving probabilistic answers. Maybe we don’t actually know the difference between those two things, we just think we do. Maybe our models are just good enough that it looks right. This is sort of what explains things like cognitive biases, and the fact that we tend to be bad at statistics. Those are showing you the ways that our model is not actually as accurate as we think it is. Now, there’s a whole sort of science question around like, how do you manage those things in the response from an LLM? But orthogonal to that, what are the use cases where this is manageable or controllable or it doesn’t matter? Where it gets tricky is when you’re using it for general search and you go to Google and you say, ‘I’ve got this pain in my stomach, is it appendicitis?’ You can’t tell what a good answer is, and it matters.
How close are they to getting the error rate down? Is it a similar thing to the path to AGI (artificial general intelligence) in that they just have no idea if or when it will arrive, or is a bit more knowable?
In principle, people have an error rate, but a very small manageable one. The debate is either, as the models get bigger and better, the error rate will shrink to the point that it doesn’t matter. Or it may be that ‘structural understanding’ will emerge. There’s a picture in my presentation of a car with two steering wheels, the point being that it doesn’t know that cars have steering wheels, it just knows that images like that have a shape like that in roughly that place. Which is why it’s wrong to say these things are lying. That’s not lying. It’s just kind of producing approximations of what the answer would look like.
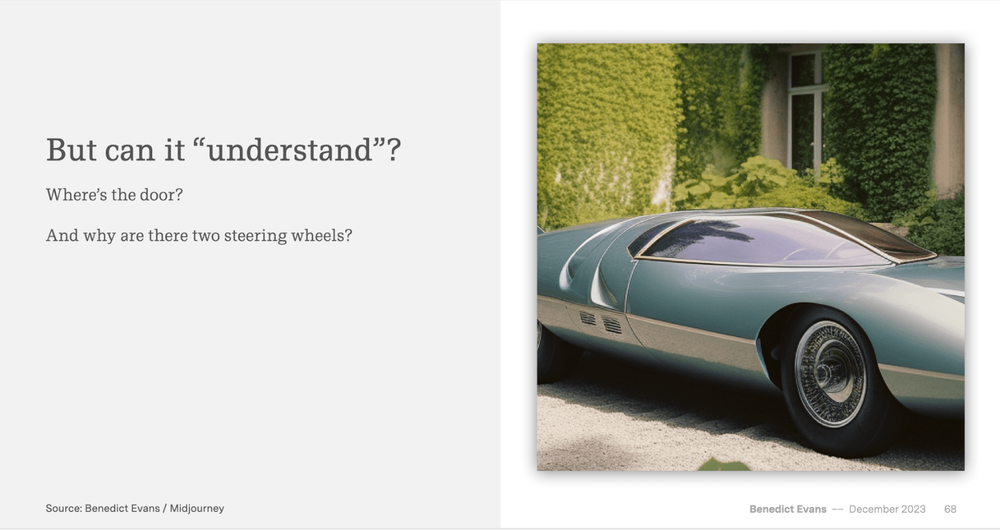
It may be that if you make the model accurate enough, then it could make a million pictures of cars, and only one of them would have two steering wheels. And it still wouldn’t know that cars never have two steering wheels, it’s just that the model is good enough that it doesn’t matter. The second possibility is that if you make the model better it will somehow know [about steering wheels] in the sense that people know that. The third answer is that this is going to flatten out and we’ll need some other theoretical breakthrough.
But we don’t know. We do not know how this is going to look in two or three years’ time. It’s very different to the iPhone where you can say, ‘the screen will get better, we’ll get 3G and they’ll put a camera on it’. We don’t have that clear sense of what is and isn’t possible here at the scientific level.
Are there any developments, announcements or watersheds that you’re keeping an eye out for in 2024 that might divine the direction that AI is headed?
There’s obviously the copyright question, which has a bunch of interesting aspects to it. But I think the operating cost on one side, the number of models, and how we think about predictability, determinism, error rates, controllability, and where you map those against what’s useful.
The quote that I use twice in my presentation is, ‘AI is anything that hasn’t been done yet’. People absolutely were thinking about databases in the 70s and early 80s as a sort of artificial intelligence. That’s what Tron is about. It’s just a database. Now you look at it like, ‘What are you talking about? It’s an ERP.’ When you do expenses, you don’t say, ‘I’m going to ask the AI if it will pay my money back’. So, by default, that’s what this will look like in 10 years’ time as well.
Benedict Evans, Analyst
Think about this. When you use your phone, the fact that every photograph you take is perfectly in focus and perfectly balanced is AI. But you don’t think of that as AI. That’s just a camera. And the fact that you can type in a word in the search of Apple Photos and Google Photos, and it will find a book in the background of a photo you took 15 years ago. Ten years ago, that was science fiction. Now, it’s just software. So by default, that’s what will happen. It will just be software. Unless, of course, it goes to AGI and kills us all.
More than half the countries on the planet will hold a national election in 2024 as people now have the ability to create realistic images and videos with generative AI. How much of a test do you think this is going to be for the technology and for democracy?
One of the interesting aspects of this has been that in the elections we had last year if a compromising video came out a politician could just say, ‘Oh, it’s fake’. So it works the opposite way as well.
Secondly, there’s no shortage of fake content; the issue is distribution. That’s what’s changed. So this is more of a challenge for Facebook and Google. If you think about what happened around the conflict in Gaza, Twitter was full of pictures that were real pictures, just not of that [conflict]. I don’t really need Midjourney to get any pictures of buildings that have been blown up. The fake bit is the description of what it is.
We’re like the moral panic carnival. All the moral panic entrepreneurs moved on from talking about how Facebook was destroying democracy to how AI is going to destroy democracy. In another couple of years they’ll have moved on to something else.
Every new technology expresses problems that society amplifies, and reshapes some channels in new ways, and this will be no different. But I would hope that people who a couple of years ago were talking about how Facebook and Cambridge Analytica destroyed civilisation are now a little bit sheepish in that we now know that it was basically a hoax.
There seems to be more impetus to learn from the mistakes made with social media and regulate AI almost from the get-go. Do you think regulators are being overly cautious?
I think there’s a question of the level of abstraction here. I always compare tech regulation to regulating cars. Cars cause all sorts of questions. Does our tax code encourage low density development? Should we pedestrianise the centre of a city? What do we think about emissions and safety? These are all real problems, but you can’t go to General Motors and tell them to fix all of them. Only two of those are engineering problems, and they’re all kind of complicated. General Motors can put seatbelts in cars, but they can’t make people wear them. So to pass a law on ‘AI’ to me is like passing a law on spreadsheets. It’s like looking at [the collapsed cryptocurrency exchange] FTX and saying, ‘Well, Microsoft screwed up because FTX had a spreadsheet.’ That's not really the right level of abstraction.
Is 2024 all about AI, as far as you’re concerned? Or is there anything that could be a key driver of change?
Part of the point of my presentation is there’s all this other shit going on. It’s like, ‘meanwhile, BYD is now selling more cars than Tesla. Meanwhile, Ikea has taken Topshop’s store in the middle of London.’
The tech industry spends a lot of time talking about what will happen in five years. Meanwhile, most agile software companies are deploying ideas from 10 years ago: SaaS [software as a service], cloud, machine learning, automation.
Somebody has come into Contagious this week and gone to some department and said, ‘We’ve just worked out something that you didn’t realise you’re wasting years of your life doing, and here is your SaaS application.’ And someone inside Contagious will look at this and say, ‘Holy fucking shit, I need this,’ and it will basically be doing Google Docs but for this thing. They will be selling you something that automates some tasks that you have not realised you are spending lots of time on, and you’ll pay them $1,000 a month and you’ll be really happy about that. Basically it will be an idea from 2005 but they’ve worked out how to market to you. That’s most software companies now. Meanwhile, the rest of the economy is being screwed up by ideas from 1995 and 2000, like maybe people will buy things on the internet?
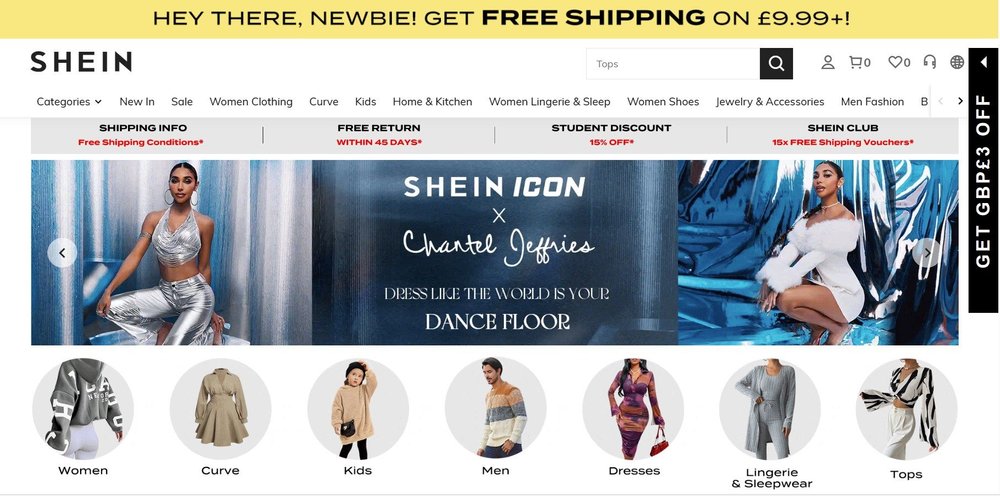
Shein is basically, ‘let’s sell fast fashion on the internet but let’s work out a new way of aggregating Chinese apparel manufacturers directly to Western consumers and shipping directly to them and have a completely different merchandising model’. You could have pitched that in 2000, except it wouldn’t have worked in 2000.
So I think that’s kind of the sequencing. All of this conversation is basically like talking about the iPhone in 2007 or 2008. Uber doesn’t exist yet, Instagram doesn’t exist, TikTok doesn’t exist. And we’re sitting here thinking, ‘Well, what would it mean if you had a device that had a camera all the time?’ I don’t think any of us were really thinking about video at that time, and the idea that everyone now has video editing in their pocket. Meanwhile, the rest of the economy carries on.
The point of that last section is, ‘Will BYD be the new Toyota?’ I don’t know. Ask a car company. What does generative AI mean for ad agencies? I don’t know. Ask Mark Read. Ask Martin Sorrell.
Want more trends and analysis? Then Download the 2023 Most Contagious Report /
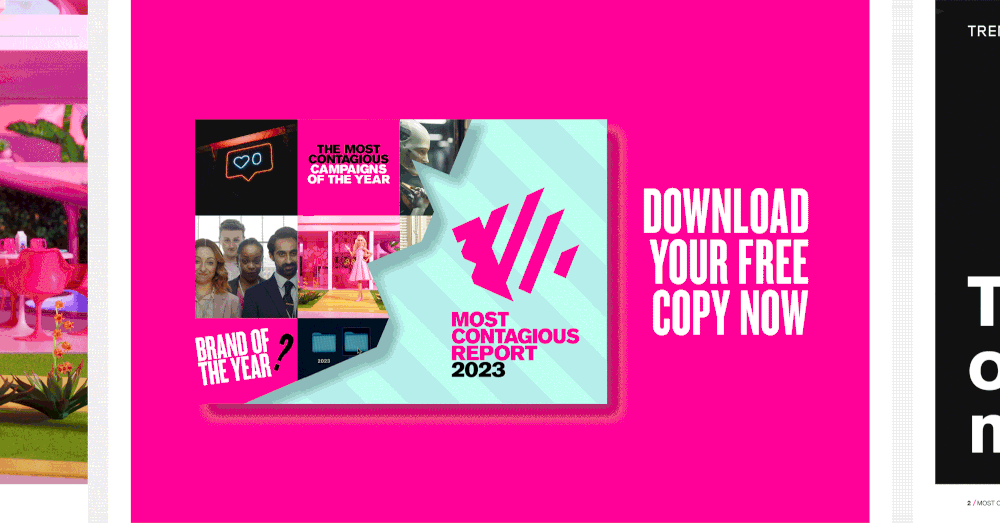
The Most Contagious Report is packed with marketing trends, campaign analyses, strategy interviews, opinions, research and even a smattering of geopolitics and culture. It’ll give you almost everything you need to hit the ground running in 2024. And it’s free (if you don’t put an inflated sense of worth on your work contact details, that is).
Want more of the same? /
We don’t just write about best-in-class campaigns, interviews and trends. Our Members also receive access to briefings, online training, webinars, live events and much more.