Daniel Kahneman & Olivier Sibony on human judgement’s hidden flaw /
Wherever there is judgement, there is noise – and probably a lot more of it than you would think. Nobel-Prize winner Daniel Kahneman and Professor Olivier Sibony discuss the unwanted variability that plagues every decision-making organisation and institution
James Swift
/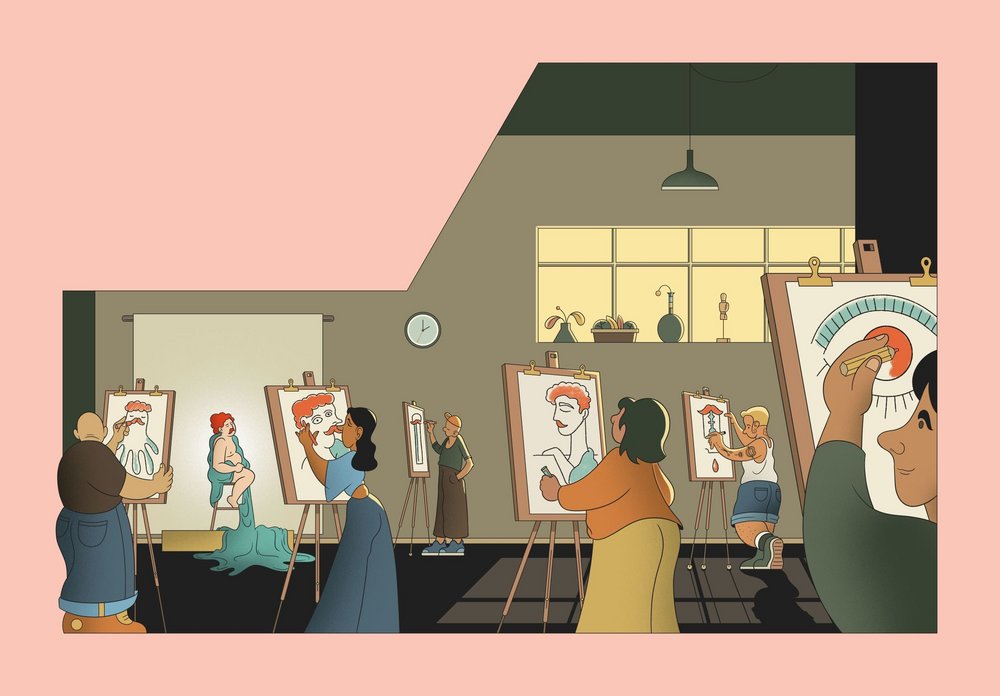
Daniel Kahneman is the preeminent authority on decision-making. His work identifying the heuristics we employ in the face of uncertainty laid the foundations upon which behavioural economics was built, and his million-selling book, Thinking, Fast and Slow, changed how people think about how people think.
No one has done more to unearth the foibles of human thought processes than Kahneman, and after almost half a century the Nobel-Prize winner is still going. Kahneman’s latest book, which he wrote with professors Olivier Sibony and Cass Sunstein (co-author of Nudge), lifts the lid on a collective delusion that plagues even our most trusted institutions and organisations.
Noise: A Flaw in Human Judgment describes the problem of unwanted variability in decision-making, ie, ‘noise’. Every day, doctors, judges, forensic scientists and other highly trained professionals make decisions that we assume are based on reason and experience. And yet, the scale of disagreement between members of the same profession when asked to assess the same problem – and even when the same person assesses the same problem at different times – is shown in this book to be scandalously high.
Contagious spoke with Kahneman and Sibony to learn more about an endemic problem in human decision-making that has been left largely unexplored until now, and to find out how you can ensure that your decisions are not overcome by noise.
What alerted you to the problem of noise?
Daniel Kahneman: I was doing some consulting with a large insurance company, and I had the idea of running an exercise where a large number of underwriters with the same highly realistic cases set dollar values on them. I also asked the executives what their expectations were about the exercise, and I asked them a specific question, which was: ‘Suppose you take two underwriters at random, by how much do you expect them to differ?’ People in general expect – and certainly those executives expected – about 10%. But the average difference between two randomly selected underwriters was about 50%, five times larger than expected. And what made that observation really interesting was that it was a complete surprise to the executives of the company. They did not know they had such a problem. And if you think about it, when you have that level of difference, you may not need underwriters at all. So it was really that combination of a great deal of noise and a problem that is unrecognised that we thought made it worth writing [about].
Daniel Kahneman
How big of a problem is noise?
Olivier Sibony: Well, it depends where you look. The general theme is, wherever you look, you are going to find noise and you’re going to find more of it than you expect. In some places where you expect not to find any noise at all because you think it’s an exact science, you’re going to find a little bit, and that’s quite troubling. An example of this would be forensic science. If you look at fingerprint experts who are looking at the same pair of fingerprints, you’ve been told to think that they are looking at the truth, and they are going to tell you what the reality is. But it’s actually not a reality, it’s a judgement. In a small but non-zero percentage of cases, they are going to disagree about the conclusions they draw from the same fingerprints.
At the other extreme, when you think that there is going to be quite a bit of disagreement, and you think that’s fair and normal, the disagreement is vastly greater than you expect. And the example of that would be HR decisions. If you ask HR executives, ‘This is the profile of the candidate, what are the candidate’s chances of success in a given position?’, you will find that some of them say zero and some of them say 100, and all the other deciles or percentiles are represented in the distribution. And when you show them the results, they look at each other in disbelief. The people who answered zero, look around, and you can see them thinking, ‘Who is the idiot who inserted 100?’ and vice versa. We literally don’t imagine how great the disagreement can be.
Daniel Kahneman
What makes human judgement noisy?
Kahneman: All biological phenomena are noisy. If the efficiency of the brain fluctuates irregularly but in a way that performance in successive moments is quite highly correlated, then across periods of time the correlation becomes much lower, so there is the spontaneous variability. And then people have different histories and their experiences are completely different, and as a result, people develop a view of problems that can be completely different, although each one of us is convinced that we are in direct contact with the truth. It’s actually called naïve realism, and each one of us is a naïve realist. We have analysed the sources of noise in detail, but the basic idea [is] that people are more different from each other than they know.
Should we be more willing to hand over our judgements to algorithms and other models?
Kahneman: In general, our answer would be yes. We should be more willing because in many situations it’s been established scientifically that algorithms and even very simple rules perform better than humans. The main advantage of rules and algorithms is that they are noise free: you present them with the same problem on two occasions and you will get the same answer. This is not true of people.
Sibony: This is not well known and it’s worth stressing, when we talk about algorithms today, people think of artificial intelligence, highly sophisticated algorithms trained on masses of data that was only developed very recently. But [there are] studies that are six or seven decades old, which established that even very basic rules, when applied consistently, will produce slightly better results than human judgements on a case-by-case basis. This is deeply counterintuitive because when we apply judgement, we make a point of being subtle, of treating each case as a separate case. If you are, for instance, hiring people, you create a story, you try to understand the personality of each person. If I tell you that a simple rule that computes three data points about each person – like their education, how many recommendations they have or whatever – would actually do a better job than you, this sounds shocking. And yet this sort of mindless consistency actually beats mindful inconsistency, which is our noisy way of thinking. That doesn’t mean of course that we should replace our decision-making systems with those kinds of very simple rules because those very simple rules are far from perfect. The improvement that they gave over our judgement is modest, and people are generally not willing to give up their right to make decisions, their sense of agency, for the fairly modest benefits that you get from those simple rules. But when you come to AI algorithms, it’s actually different because now the gain is much larger.
Kahneman: The quality of prediction in general is usually low because the world is largely unpredictable. We have in the book a concept that we call ‘objective ignorance’, which is that the most that you can know in many situations is very little, and the problem of judgement and the problem of prediction is extracting all the information you can – although when you have extracted all available information you will still not be doing very well. So the algorithms are very far from perfect. People have the idea that AI is perfectly valid. It is not and it cannot be because the future is unpredictable. It is just more accurate than human versions, and that is something that people haven’t appreciated.
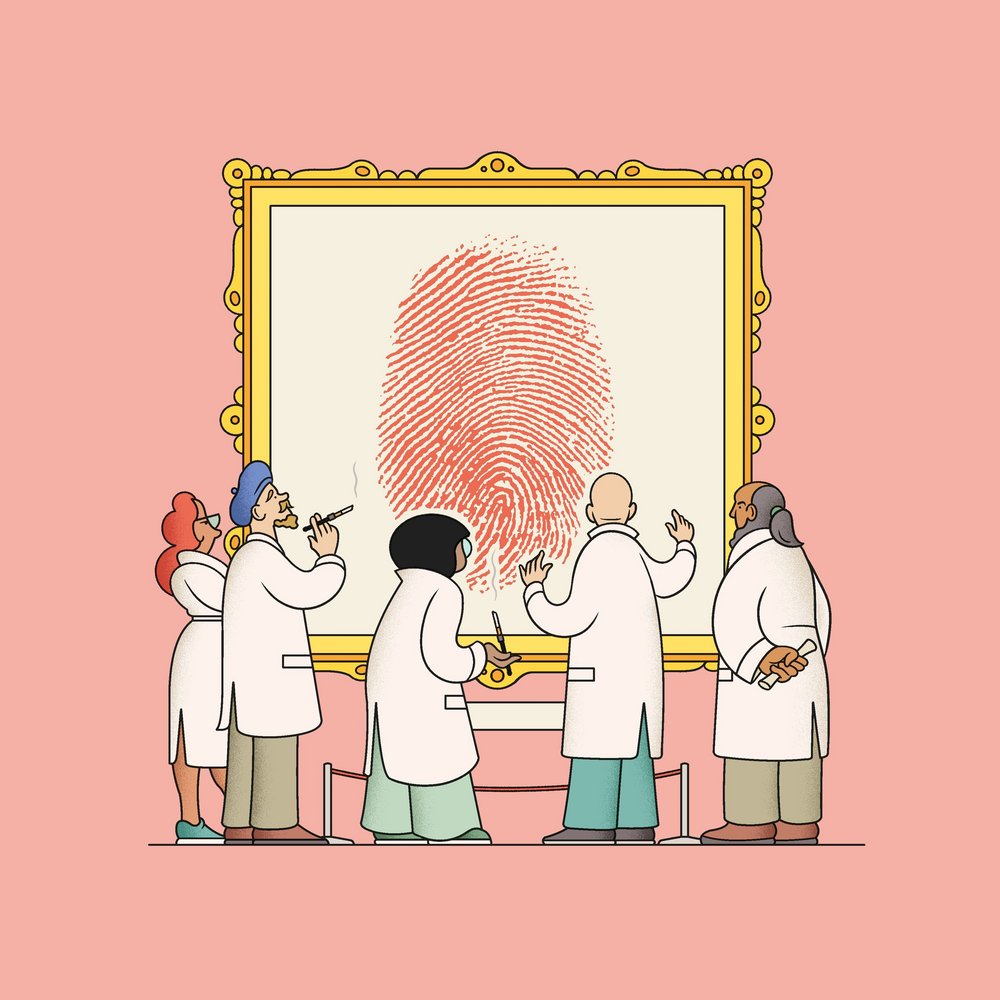
Olivier Sibony
So there are no situations that you’ve come across where human judgement outperforms simple models or AI?
Kahneman: It’s a tie at best.
Sibony: We talk a lot about AI here, but the main point we make in the book is not that we should replace all human judgements with machines and algorithms. The reason we are not making that point is that, first, for many important decisions, it’s impossible because we don’t have data or it’s not easy to code, etc. Second, even when it is possible, it’s quite often not desirable, either because decision-makers resist the position, or because the recipients of those decisions want to deal with a human. You want a human doctor, you want a human judge if you are on trial. There is an important process dimension to this. And in all those situations our recommendation is not to replace humans with machines – although it theoretically might be an improvement in some situations – it’s that we actually try to make human judgements a little bit better by making them a bit less noisy. And that’s what we call decision hygiene.
Kahneman: And the trend is that AI is becoming increasingly acceptable. I heard that there is a province in China where they have been experimenting in the bankruptcy court with decisions being made by AI, and they’re being made, of course, very much faster, and they’re being made better and people are satisfied with the answers.
Sibony: If they weren’t satisfied, I’m not sure we would hear about it...
Daniel Kahneman
I believe you identified stable pattern noise as the biggest culprit of noise. What is that and what can be done about it?
Kahneman: What it is really is that people see the world very differently. So for example, in the case of judges, there are two sources of noise that are immediately apparent to people: level noise, some judges being more severe than others; and occasion noise, which is that on different occasions the same case may evoke different responses depending on the judge’s state of mind. But the really important and interesting source of variability is that when judges are presented with a series of cases, they will not rank them by severity in the same way. And that is what we call pattern noise. There is someone who is really shocked when he sees a crime where the victim is an old person, there is someone else who is shocked by the mistreatment of women or the mistreatment of children, or in some cases, mistreatment of cats. People have vastly different sensitivities. And it’s not only judges, it’s any topic that requires judgement. That’s because judgement is really the integration of a large amount of information: it’s an informal process of integration, which is what distinguishes judgement from computation. In the process of judgement, the weight that people give to different items, or the way they combine items, is quite different.
Sibony: The paradox here is that everything that he just said – about our judgements being different because our histories are different, our personalities are different, our values are different – is usually celebrated as good news, as the fact that we’re all individual and unique, and therefore creative and so on. And it’s of course true that no one would want to live in a world of clones. But paradoxically, we can’t have it both ways. We can’t expect people in a judgement setting, as we describe it (which means in an organisation where you’re trying to get as close as possible to the correct answer to a question) to produce identical answers, or even nearly identical answers. We need to recognise that in some situations diversity is wonderful and in other situations it’s actually problematic. The fact that people have different views is not always good news. If you go to the doctor and he tells you, ‘You have this,’ and then you go to another doctor and he tells you something completely different, you don’t say, ‘Oh, how wonderful, we’re being creative here,’ you say, ‘There’s a problem.’
Earlier you mentioned decision hygiene and in the book you suggest several other ways to deal with noise. Is there one method of reducing noise that is most important?
Kahneman: There are some that are easier to apply than others, and the one that is easiest is to turn your judgement from absolute to comparative. Try not to evaluate cases on their own but to rank or compare cases to each other or to past cases on a scale. That’s one simple recommendation. The deepest recommendation we have is [to] break up problems: make a plan for how you’re going to reach [a judgement] and break it into parts. Make the parts independent from each other. Valid aggregation of information requires the information to be independent, and that applies at any level. You don’t want to form a global impression before you have formed specific impressions of particular aspects of the problem. Our main recommendation would probably be: plan which attributes are the problem you are going to evaluate separately before you try to reach a final judgement, and delay the final judgement until all that information is in. The danger with intuition is primarily when it’s premature.
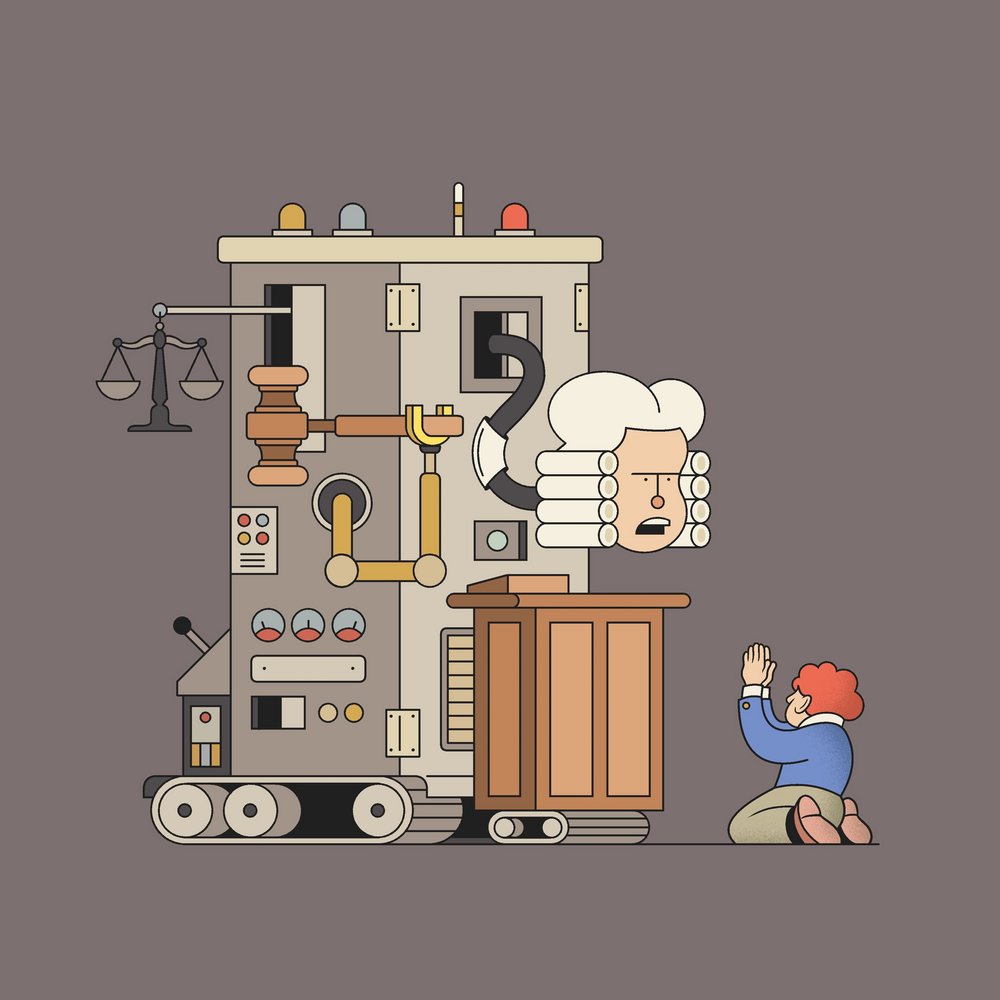
Finally, how do you distinguish noise from bias?
Kahneman: When we speak of noise and biases, we speak of judgement noise and judgement bias, and we speak of them in very strict analogy to measurement noise and measurement bias. What is measurement noise? It refers to the fact that if your scale is fine enough and you make repeated judgements of the same object, you’re not going to come up with exactly the same number. That variability is noise, and the average error that you make, the systematic tendency of errors to overestimate or underestimate that truth, that is statistical bias. Measurement noise and measurement bias are very clearly defined. And we define judgement noise and judgement bias in strict equivalence. Now, it turns out in the theory of measurement that noise and bias have similar weights conceptually. That’s given in the formula for global inaccuracy, in the square of the bias plus the square of the noise. So, in that formula they’re really interchangeable mathematically [but] have quite different rules. For example, by aggregating separate judgements, you are guaranteed to eliminate noise, but you do not reduce bias. So, the wisdom of the crowd is very poorly named because crowds are guaranteed to eliminate noise, but they’re not guaranteed to approach the truth. They only approach the truth when there is no bias. In the conversation about error, both the scientific conversation and the public conversation, error is typically associated with bias. What we are trying to add here is that there is a neglected component of error.
Sibony: There’s also the misconception that noise doesn’t matter because on average, it cancels out. And it is true that, by definition, noise is an error that averages zero over many cases, but of course the old jokes about averages being misleading apply here. If doctors on average diagnose the correct number of diseases, but they treat people who are not sick and fail to treat people who are, then that’s a problem. If you price your insurance policies sometimes too high and sometimes too low with an average that is correct, you’re making two costly mistakes. So noise does matter because we don’t live in a world of averages; we live in a world where each judgement matters.
Want more of the same? /
We don’t just write about best-in-class campaigns, interviews and trends. Our Members also receive access to briefings, online training, webinars, live events and much more.